
Is Machine Learning the Future of Fleet Technology?
In this week’s episode of Fleet FYIs, I’m joined by Utilimarc product manager, Lanelle Duke. Lanelle has an extensive background in product management and development, and is currently working as part of Utilimarc’s product development team. She has specifically been a product manager for the previous eight years, four of which were spent in a deep dive with data analytics and data visualization.
Today, Lanelle and I open up a conversation surrounding machine learning, AI and algorithms – and their affect on the fleet industry. The fleet industry is in a unique position, having seen other industries begin to adopt this technology, and now adapting it to suit their operations and management strategies.
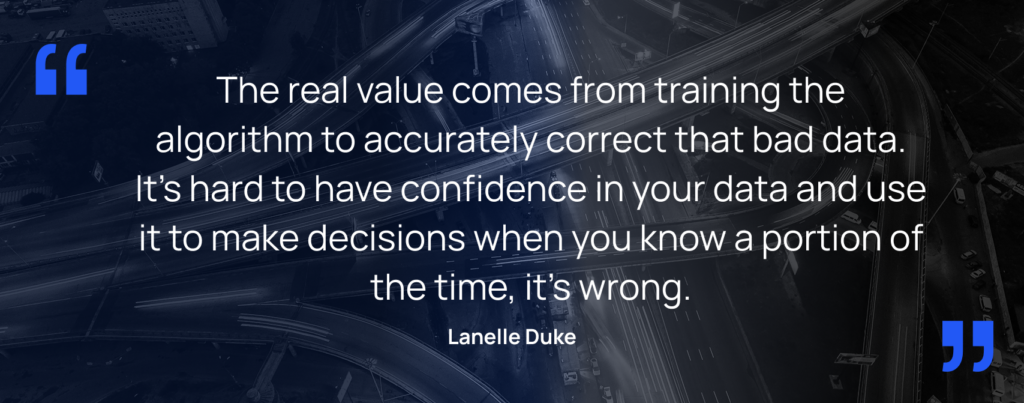
Here’s a summary of my conversation with Lanelle:
With so much going on surrounding machine learning and artificial intelligence technology being injected into a plethora of industries globally, Lanelle and I went into a brief into – as there was no way that everything could be covered in one episode. Here were some of the key takeaways:
- Machine learning is affecting nearly every industry on a global scale, and the fleet industry is in a unique position to take advantage of this technology.
- Utilizing data scientists on your team (or simply making sure you have one on your team) is one of the best decisions you can make for your fleet management strategy.
- TEST. Always test – whether it’s your data, a new product or operational processes.
and - How preventive and predictive technology can be integrated into fleet management alongside artificial intelligence.
Some of Lanelle’s most memorable quotes from this episode:
“It’s hard to have confidence in your data and use it to make decisions when you know a portion of the time, it’s wrong.”
Lanelle Duke, Is Machine Learning the Future of Fleet Technology? An Intro to AI with Lanelle Duke | Utilimarc Fleet FYIs Podcast
“Having a data scientist on your team is an incredible advantage. If he or she is able to develop an algorithm that can solve that problem, a product manager can then figure out how to turn that algorithm, and whatever that output is it produces, into a brand new product or a set of product features for an existing product, that customers can use and get a lot of value from.”
and
The other major contributor is the internet of things. Many everyday objects like a refrigerator or a car or a telematics device is transmitting data which is collected and stored, and is now made available for advanced analysis. We didn’t have all those kinds of devices transmitting data in the past. There’s just so much now, it’s so prevalent, and it’s just going to expand.

Is Machine Learning the Future of Fleet Technology? An Intro to AI with Lanelle Duke | Utilimarc Fleet FYIs Episode 4
Gretchen Reese (00:04):
Hey there. Gretchen here. Welcome to Fleet FYIs, the weekly podcast by Utilimarc that makes fleet management strategy smarter by bringing to you nearly two decades’ worth of data insights, industry hot topics, and expert analysts together in conversation. Our aim is to help you better understand your data and your key metrics by hosting candid conversations with some of the industry’s finest. But before we begin, if this is your first time listening to our podcast, thanks for hitting the play button. I’m so glad you decided to come along for the ride and have a listen.
Gretchen Reese (00:36):
Once you finish today’s episode, if you could take a few minutes to leave us a review, we’d really appreciate it. Give us a rating, tell us what you liked, or perhaps what you didn’t, or you can leave us a comment or a question about what we’ve covered today. Also, if you have a topic that you’d love for us to cover but we haven’t touched on yet, let us know. We’d be happy to go over it in detail in a later episode. Sound good? All right, let’s get back to the show.
Gretchen Reese (01:05):
Hey guys. Welcome back to another week of Fleet FYIs. I hope you’re having a wonderful week and are hopefully looking forward to an even better weekend. I want to start today’s episode off with a bit of a confession. My search history has been full of one topic lately, and it’s been one that’s fascinated me for a while. In short, today we’re talking about machine learning. And to kick things off for this episode, I’d love to jump into a quick look and a short intro about how the fleet industry is actually moving forward with artificial intelligence, better known as AI, machine learning and algorithms as a whole.
Gretchen Reese (01:43):
Now, this isn’t new technology. It wasn’t invented yesterday, and it’s not going to take over the world tomorrow. But nearly every single industry is introducing this technology into their day to day operations, trying to make things easier for their people, and trying to make things repeatable and easily recognizable if they’re looking for patterns for the future. Now, they could have been early adopters or slow burners, and I’m not really here to say one way or the other which one was better or worse. I mean, because sometimes it’s just worth seeing if something works first before you jump all in with two feet. It makes sense.
Gretchen Reese (02:23):
But what I’d like to look at today is the unique position that the fleet industry is in with AI, machine learning and algorithms. Some of the questions that I’ve been asking, not just Googled in the past few months, but just over the years that this has been introduced, is how can these technologies make for smarter management strategy? Or relatable to the fleet field, how can it make for smarter fleet management strategy? How can it predict maintenance or prevent certain events from happening? There are so many questions that I’ve got, and I’d like to bring someone in to help me dig a little bit deeper into these questions and this topic as a whole.
Gretchen Reese (03:01):
So I’d love to introduce you to today’s guest. Her name is Lanelle Duke. She’s had an extensive background in product management and development, and is currently working as part of Utilimarc’s product development team. She has specifically been a product manager for the previous eight years, four of which were spent in a deep dive with data analytics and data visualization. And prior to that, she came from the world of marketing. So I think that she’ll have a really interesting perspective when it comes to this newer technology and how it’s being introduced into not just the fleet industry, but industries worldwide. So if you’re ready, because I am, I’d love to bring her on.
Gretchen Reese (03:42):
Hi, Lanelle. Welcome to the podcast. I’m so glad you were able to join me today.
Lanelle Duke (03:47):
Oh, thanks for having me. I’m excited to be here.
Gretchen Reese (03:50):
It’s an absolute pleasure, as I’m sure you know. And I’d love to jump into it straight away. So I’m sure you know this, but we’ve been seeing machine learning and AI become a large part of nearly every industry. And for the industries it hasn’t touched yet, it probably will very soon. Can you start us off with telling me, in what ways have you seen this begin to affect the fleet industry today or over the last couple of years?
Lanelle Duke (04:16):
Well, Gretchen, you’re correct that data science techniques like artificial intelligence and machine learning are becoming pervasive to pretty much every industry. I saw the financial industry become an early adopter, as was supply chain management, advertising, and also you see a lot of the large retailers using it. The fleet industry is exploring how it can apply these same approaches and realize the gains that other industries have been experiencing, just putting their own twist on it for fleet.
Lanelle Duke (04:48):
Specific to the fleet industry, where I’ve seen machine learning used successfully is to improve data quality. An algorithm can be trained to recognize patterns in data, to analyze and categorize data based on a good clean data set. So once it’s seen many, many examples of what good data looks like, it can identify when it is shown bad or inaccurate data. And that’s really the first step, is training the algorithm to identify the bad data points.
Lanelle Duke (05:18):
The real value comes from training the algorithm to accurately correct that bad data. It’s hard to have confidence in your data and use it to make decisions when you know a portion of the time, it’s wrong. So being able to employ very highly accurate algorithm eliminates that worry and will allow a fleet manager to use their data to its full potential, to make decisions on an operational level or on a strategic level.
Gretchen Reese (05:46):
Absolutely. So when you’re saying bad data, how long do you think it would take for an algorithm to be able to identify what you called a bad data point?
Lanelle Duke (05:57):
It’s pretty much immediate.
Gretchen Reese (05:59):
Oh, wow.
Lanelle Duke (06:00):
It’s so fast. Just the speed of the servers and things today, it’s just really fast, and it’s immediate. It happens automatically. It’s seconds or sub seconds.
Gretchen Reese (06:15):
Wow. That’s really impressive. So have you been seeing any trends emerging on the product creation side, whether it’s for fleet or maybe in your past experience?
Lanelle Duke (06:27):
Yeah, absolutely. A main part of my job as product manager is to listen to my customers to learn key pain points regarding data, and understand how it negatively impacts them. So what are their challenges? What are the frustrations? What’s preventing them to do their job? Sometimes when talking to customers, you can find a large industry problem that hasn’t been able to be solved by traditional approaches. That’s why having a data scientist on your team is an incredible advantage. If he or she is able to develop an algorithm that can solve that problem, a product manager can then figure out how to turn that algorithm, and whatever that output is it produces, into a brand new product or a set of product features for an existing product, that customers can use and get a lot of value from.
Gretchen Reese (07:22):
So do you think, then, if, say, for example, you’re updating a product with new features, what would be some of the pain points that would lead you to most likely develop said new features with maybe a machine learning component for an existing product?
Lanelle Duke (07:39):
I think if it’s something that’s pervasive, if I hear a one-off thing from a customer of, “This as a challenge for me, this is a struggle,” and I just hear it once, then I may be less likely to act on it. If I have 10 or 20 of my best customers express that same concern, then that tells me, that’s kind of a red flag to me to tell me that that’s something that is a real pain point. It’s something that if all these big customers are all grappling with it, and no one’s been able to come up with a solution yet or solve it, and then just our business in general hasn’t been able to solve it with traditional approaches.
Lanelle Duke (08:19):
If it’s something that’s based in data and you can apply machine learning or a neural network or artificial intelligence to it and solve it, then that’s where you get the real value. So that’s really what I’m looking for, is I’m looking for problems that I hear a lot that seem pervasive and that seem like maybe there’s something that my specific product could do, using data science, to fix it. Those things I get really excited about.
Gretchen Reese (08:49):
So with AI and machine learning, it’s all about predictive technology, and basically a machine being able to predict if, like you said, you see a bad data point, or maybe being able to help you solve a problem that you might be having. How do you think having a technology oriented view will help make fleet management more predictive? Is that something that they would be able to use day to day, perhaps with fuel costs, budget setting, or maintenance?
Lanelle Duke (09:17):
Yeah, I think there’s actually huge potential in the fleet industry to use data science techniques to predict future outcomes. And there are some things there that are very costly and very expensive. So if you could solve it, it would have a major impact for pretty much any organization no matter what size. I think in the future, a fleet manager will use data science to identify drivers who might be more likely to get into an accident in the next 30 or 60 days. Say you had 10,000 drivers that were driving your company vehicles, and you knew the 25 that might be more likely than the rest to get into an accident, that’s really helpful to know.
Lanelle Duke (10:04):
You could put training in place, you could do outreach, you could put in processes that might mitigate that risk. And I think when we’re talking about prediction, that’s something that would be highly effective and would save a lot of money and be really helpful. And then I think another area where you’ll see prediction really evolving and coming into the forefront is when a vehicle is likely to break down. If you have 10,000 vehicles on the road, and they’re all out doing their jobs and they’re servicing customers, drivers are getting work done, which of those might be likely to break down next week? That would be really helpful to know, and why, so that you can pull them off the road and fix them. So knowing, dealing with that kind of thing in advance will help to eliminate fire drills where you might have to find a replacement vehicle to fill in very quickly to maintain your service levels.
Gretchen Reese (11:08):
No kidding. Because I feel like having those fire drills, they’re very stressful. I mean, ask any fleet manager, I’m sure they’ll tell you the same thing. But aside from maintenance, taking that out of the equation, what do you think in terms of departments, which departments do you think will see the most benefit from introducing predictive technology or having that even just being injected into their fleet management strategy?
Lanelle Duke (11:33):
I think the obvious one is the fleet team. I think they will benefit the most, just on many levels. They will be probably one of the key groups that will be so much more enjoying their job to have this kind of information at their fingertips and use it make decisions in real time. I think potentially another department that might benefit would be procurement department, because a lot of times they’re involved in acquiring new vehicles or new kinds of service contracts, service providers, parts, things like that.
Gretchen Reese (12:15):
So Lanelle, I’d love to get your opinion on this. What would you think would be the primary difference between preventative and predictive fleet software technology, just as you’ve seen it in your experience with product development design or even now today?
Lanelle Duke (12:31):
In my mind, I think preventive is more about PMs, right, preventive maintenance. It’s bringing a vehicle in for service or replacing a part based on some cadenced schedule that’s being followed. So you’ve put your schedule in place, and when it hits a certain mileage threshold, it’s coming in whether it needs it or not. And in practice, that sounds like a great approach, and it really is, right? That’s a great way to go. It’s very methodical. It’s very logical, so it’s good. But I think predictive really takes it up a notch.
Lanelle Duke (13:09):
What if you only brought your vehicles in for service or replaced parts when it was really needed, right? Like maybe it doesn’t need a certain kind of PM at 10,000 miles. Maybe it could go to 12 or 15. So if you were using more of a predictive kind of a schedule and machine learning or data science techniques to figure out when you need to bring a vehicle in, that could save you a lot of money, and it could eliminate some of the disruption of taking a vehicle off the road and putting it in the garage, and then needing to find a replacement. So you said in my opinion, and that’s my opinion.
Gretchen Reese (13:52):
So tell me, then. How can predictive or even preventative fleet management software tools work hand in hand, say for example, with a BI platform for a more robust fleet management strategy? I mean, you mentioned data visualization, but how do those two things pair together?
Lanelle Duke (14:11):
I think in the fleet industry, there are a lot of different data sources that can be leveraged. And most people, or most organizations have a fleet management system, which is usually the backbone of how they’re managing their assets and knowing when they need service, and all of that. There’s telematics, which a lot of fleets have. Fuel is another big component. Sometimes weather or traffic, or sometimes information out of the company’s HR systems have some valuable insights. So I guess the point is, there are some common data sources that that can be leveraged. There’s also probably some interesting things that could also be combined that aren’t the sorts of things you see for data in the fleet industry. I think the challenge that anyone who works in fleet understands very well, and it’s not a surprise, is that this data is siloed.
Lanelle Duke (15:09):
It’s very difficult to bring it all into one environment so you can do something amazing with it. And I think that is the future. It’s integrated key fleet data sets mixed in with other kinds of data sets that provide value and interest. And then the trick is having the knowledge and expertise and time to be able to do something with it. Predictive being something that would be really exciting. What could you do if you had all that together? I think the possibilities are endless.
Lanelle Duke (15:43):
So when you talk about accident prediction and prevention, again, as we were just talking about a little bit earlier, when a company vehicle gets into an accident, that’s very costly. There’s the repair costs that can be very large. There’s the potential for a lawsuit. Insurance costs could go up as a result. There’s damage to the company’s brand reputation potentially. And then also, as you mentioned before, the fire drill it creates in needing to find a replacement vehicle immediately. So just in that one example, if you could solve for that scenario, it would be a huge benefit for a fleet manager or a fleet team and a huge cost savings.
Gretchen Reese (16:24):
So do you think a BI platform, perhaps that might be the key to this, like you said, a future of fleet tech?
Lanelle Duke (16:32):
I absolutely do. I can’t wait.
Gretchen Reese (16:36):
So Lanelle, as you know, this was a very quick intro. It was by no means everything that can be said about machine learning, as there’s just so much more to touch on, but is there anything else that you’d like to add for our audience before we wrap this episode up?
Lanelle Duke (16:52):
I just have just a little bit more to say about it, just to kind of set up the whole thing about machine learning and where it’s going and why. And I think in recent years, we’ve seen a rapid acceleration in the use of machine learning and other data science techniques. You’re also seeing universities offering degrees in data science and data engineering, and those are very new and exciting career choices. And as a result, or there’s been some contributing factors which have enabled this.
Lanelle Duke (17:25):
So Cloud storage is very cheap. You can rent servers today that you can spin up or down as you need them instead of having to make that costly investment yourself. The other major contributor is the internet of things. Many everyday objects like a refrigerator or a car or a telematics device is transmitting data which is collected and stored, and is now made available for advanced analysis. We didn’t have all those kinds of devices transmitting data in the past. There’s just so much now, it’s so prevalent, and it’s just going to expand. So I think combining all that together, we’re at the very beginning of something that’s very exciting, and I’m really interested to see where we’re going to be with this 10 or so years from now.
Gretchen Reese (18:16):
Yeah, absolutely. I think it’ll be really interesting just to even see how it starts to play out even over the next one to two years, because I think this is fast moving, so you’re absolutely right. So just in case, Lanelle, our audience wants to continue this conversation perhaps with you offline, is there anywhere that they would be able to find you outside of contacting Fleet FYIs?
Lanelle Duke (18:41):
Probably the best is my LinkedIn profile. I am out there on LinkedIn, and that’s a great way to get ahold of me.
Gretchen Reese (18:49):
All right. Well, again, Lanelle, thank you so much for joining me on Fleet FYIs today. It’s been great to have you, and I really appreciate it.
Lanelle Duke (18:56):
You’re welcome.
Gretchen Reese (18:57):
All right. So that was just a quick intro to the addition of machine learning and artificial intelligence or AI into fleet management strategy and the fleet industry as a whole. And I really think that machine learning is going to be an interesting part of fleet management as we move forward into 2021, the next five years, and even later on down the line. I think it will be certainly interesting to see how the use of this technology evolves, as well as how the technology itself evolves, because it’s still changing. It’s not set in stone, and there’s always going to be the latest and greatest coming out every year or even more frequently than that.
Gretchen Reese (19:37):
I’d love to hear from you, though. How do you think that machine learning and AI will begin to shape or have already begun to reshape the fleet industry? Is this technology something that you’re looking forward to? Share your response with us using the hashtag #UtilimarcFleetFYIs, because I’m really keen to see some more opinions surrounding this topic and just wanting to build out the conversation.
Gretchen Reese (19:58):
But before I sign off for the day, I just want to take this opportunity to ask that if you liked this episode, to please leave us a review because every single review helps us out tremendously, and it also helps more fleet folks just like you find our podcast and engage with it as well. We’ll be back again next Thursday, but until then, you can find us on our website, www.Utilimarc.com, spelled out U-T-I-L-I-M-A-R-C, dot com, as well as on our social media platforms on Instagram, Facebook, and LinkedIn by searching the user handle @Utilimarc. Until next time, this has been your weekly dose of Fleet FYIs, and I’ll catch you later.
If you or someone you know is interested in being a guest on Fleet FYIs, please email our content manager with your request.